Guide to Choosing an LLM: 2024 Edition
2024-09-26
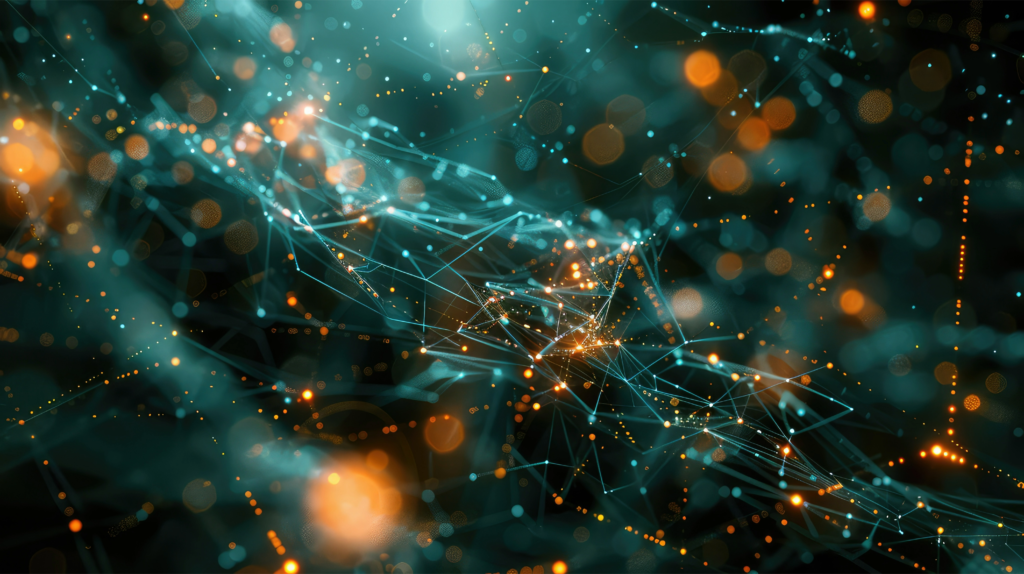
目次
1. Executive Summary
Open-source Large Language Models (LLMs) are rapidly closing the gap with closed-source LLMs, with the performance difference shrinking by the day. When choosing an LLM, companies need to consider not only performance but also factors such as thespeed of innovation, customization potential, transparency, support structure, andCostThis article explores the differences between open-source and closed-source LLMs and explains why open-source models are becoming a clear choice for many businesses.
2. Background and Current Analysis
2.1 Background
Advancements in AI technology have led to rapid progress in the development and application of Large Language Models (LLMs). Open-source LLMs, with theirAccessibilityandrapid evolution driven by community contributions, have become strong competitors to their closed-source counterparts.
2.2 Current Trends and Challenges
Open-source LLMs are continually improving in performance, and companies are placing increasing importance not only on performance but also on factors such ascost efficiencyandCustomizability, andTransparency. On the other hand, while closed-source LLMs often offer high performance, they come with challenges such asexpensive licensing feeandlimited customizationoptions.
3. Main Content
3.1 Detailed Analysis of the Main Theme
Comparison of Open-Source and Closed-Source LLMs
Prospectives | Open-Source LLM | Closed-Source LLM |
---|---|---|
Accessibility | Available without restrictions | Usage is often restricted |
Innovation Speed | Rapid, driven by community efforts | Limited to in-house developments |
Customizability | High customizability | Customization options are limited |
Transparency | High transparency with open code | Low transparency, algorithms are closed |
Support | Community support | Dedicated customer support |
Cost | Low cost, predictable | High cost, requires licensing fees |
Figure 1: Comparison Table of Open-Source and Closed-Source LLMs
Accessibility
Open-source LLMs are freely accessible to everyone for use, modification, and development, promoting the democratization of AI. They allow for code verification and improvement by the entire community, enabling quick issue resolution and feature enhancements. In contrast, closed-source LLMs are only available within specific companies or organizations, with technical details kept confidential. This restricts the speed and flexibility of development.
Speed of Innovation and Customization
Open-source LLMs enable rapid innovation through collaboration among numerous developers. Companies can customize these models to meet their specific needs and add new features. On the other hand, closed-source LLMs tend to have a slower innovation pace due to limited development resources and offer restricted customization, which often leads to vendor dependency.
Transparency
Open-source LLMs, with their open codebase, offer high transparency, allowing users to understand the internal structure of the models. This transparency increases reliability and facilitates better risk management. Closed-source LLMs, however, do not disclose their algorithms or data, making it difficult for users to fully comprehend the model's behavior. This lack of transparency can lead to potential risks and concerns about reliability.
Support
Open-source LLMs are backed by community-based support. While this approach provides diverse perspectives and solutions, it may lack consistency. In contrast, closed-source LLMs offer dedicated support teams, providing prompt and professional assistance. However, the cost of such support can be quite high.
Cost Efficiency
Companies leveraging open-source LLMs can fine-tune models using their own data to meet specific industry needs. For example, in the healthcare industry, open-source LLMs are being utilized to develop models that align with specialized terminology and regulations, enhancing diagnostic support and patient communication.
3.2 Business Application Cases
Companies leveraging open-source LLMs can fine-tune models using their own data to meet specific industry needs. For example, in the healthcare industry, open-source LLMs are being utilized to develop models that align with specialized terminology and regulations, enhancing diagnostic support and patient communication.
3.3 Technical and Organizational Challenges
Technical Challenges
・Data Quality : High-quality training data is essential.
・Model Bias : Verification is needed to build unbiased models.
・Implementation Cost : Initial setup and infrastructure costs may be significant.
Organizational Challenges
・Talent Acquisition : Specialists with advanced skills are needed.
・Internal Process Development : A framework for model operation is necessary.
・Vendor Lock-In Mitigation: A strategic plan is needed for transitioning away from closed-source solutions.
4. Future Outlook and Strategic Insights
4.1 Future Potential and Business Impact
Open-source LLMs are expected to continue improving in performance and expand their communities, further promoting the democratization of AI. This trend enables companies to develop innovative solutions at a lower cost, thereby enhancing their competitive edge in the market.
4.2 Action Plan and Recommended Strategies for Companies
Step 1: Evaluation and Selection
Assess and select an open-source LLM that aligns with the company’s needs.
Step 2: Establishing a Technical Foundation
Secure the necessary infrastructure and personnel for implementation.
Step 3: Model Customization
Fine-tune the model with company-specific data to optimize its performance.
Step 4: Risk Management
Implement measures to address security and ethical concerns.
Step 5: Continuous Improvement
Collaborate with the community to continuously update and optimize the model.
5. Conclusion and Key Takeaways
Open-source LLMs offer superior customizability, transparency, cost efficiency, and innovation speed, making them an attractive option for many companies. The key benefits include:
Customization and Flexibility: Models can be tailored to meet specific business needs.
Cost Reduction: No licensing fees, enabling AI implementation within budget.
Transparency and Reliability: Open-source code ensures the model's reliability and trustworthiness.
Community Support: Leverage the power of a global community of developers to adopt the latest technologies.
By strategically adopting open-source LLMs and leveraging these advantages, companies can better adapt to the future business landscape and establish a competitive edge.
Source
・Arcee.ai社「How to Choose Between Open Source and Closed Source LLMs: A 2024 Guide」
About NITI
Our mission is to deliver cutting-edge technology swiftly and bring innovation to the real world. We tackle various real-world challenges both domestically and internationally with top-tier AI technology.
Our mission is to deliver cutting-edge technology swiftly, innovating in the real world.
We will use the latest AI technology to solve various real-world problems both in Japan and overseas.
Why Not Start with a Casual Consultation?
Why not come and have a chat with us? Talking can broaden your perspective and help you see future actions and solutions. Please feel free to contact us.
Would you like to have a casual brainstorming session with us?
Talking things through can broaden your perspective and help uncover future actions and solutions. Feel free to reach out to us anytime.
Please feel free to contact us.